Automated Model Training Pipeline v1.0
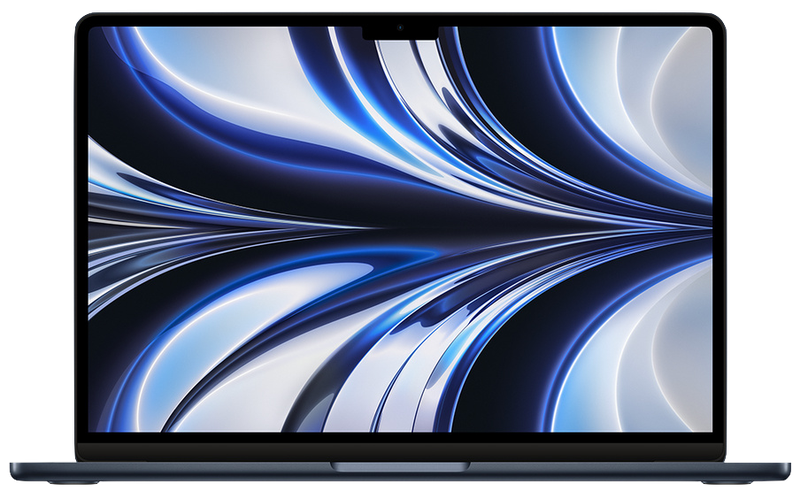
About the project:
In this project, I addressed a bottleneck in our data science workflow that was significantly limiting the data science team's productivity. Previously, our data scientists would manually launch an EC2 instance and set up a machine learning model for training. This was a time-consuming process - typically it took several hours for a data scientist to train a single model. Evaluating the results of this process usually led to a throughput of just one model per day per data scientist.To optimize this process, I developed an API using FastAPI with Python. The API is integrated with the Kubernetes API to automatically launch an EKS job for training a specified model pipeline. This innovative approach allowed us to initiate multiple model training runs via the API. The model pipeline to be trained could be easily specified through distinct configuration files, enabling us to train a variety of models simultaneously. As a result, we significantly increased our model training capacity and reduced the time spent per model, with the only limitation being the resource constraints of our Kubernetes cluster. We could launch at most 50 training runs on a test dataset. Through this project, we were able to greatly enhance our data science team's productivity and efficiency.
Technology used:
Python, Kubernetes, MLFlow, FastAPI, AWS, Docker